As increasing amounts of economic, entertainment and social activities are occurring using native and web applications, it has become essential for developers to analyze user interactions in order to better understand their behavior and increase engagement and monetization. In this paper, we describe how JumpStart, a real-time event analytics service, utilizes machine learning techniques for empowering developers and businesses to both identify users exhibiting similar behavior and discover user interaction patterns that are strongly correlated with specific activities (e.g., purchases). Discovered interaction patterns can be used for enabling contextual real-time feedback via JumpStart’s complex event pattern matching.
Machine Learning Techniques for Mobile Application Event Analysis
Machine Learning Techniques for Mobile Application Event Analysis
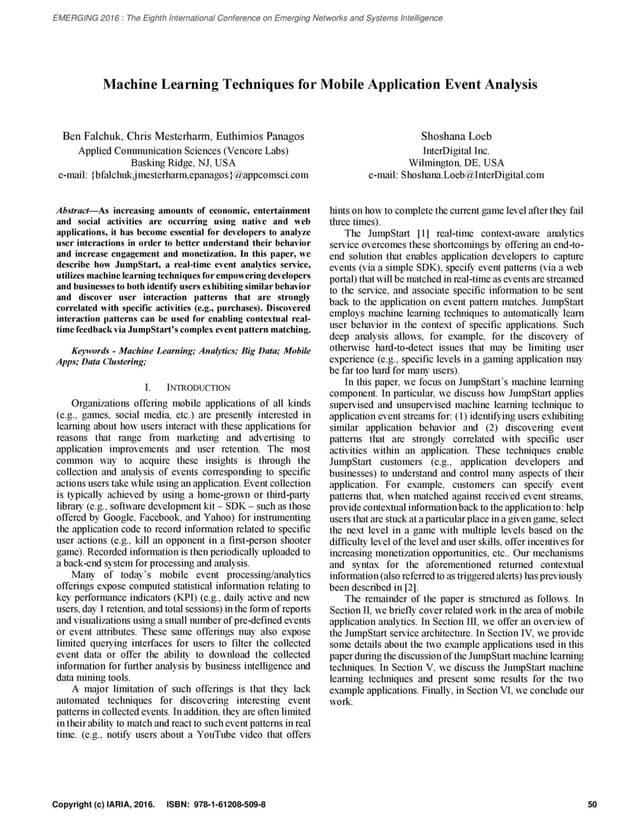
Machine Learning Techniques for Mobile Application Event Analysis
Research Paper / Dec 2016
Related Content
White Paper /May 2025
Media over Wireless: Networks for Ubiquitous Video
Research Paper /Mar 2025
To realize the objectives of Integrated Sensing and
Communication (ISAC) in 6G, there is a need to introduce
new functionalities in 6G core (6GC) architecture that are
dynamic and resource-efficient. In ISAC, sensing signals are used by a Sensing Receiver (SRx) to measure and report Sensing Data Points (SDPs) to the network. However, a direct approach involving …
Research Paper /Mar 2025
This paper proposes a method that enhances the compression performance of the current model under development for the upcoming MPEG standard on Feature Compression for Machines (FCM).
This standard aims at providing inter-operable compressed bitstreams of features in the context of split computing, i.e., when the inference of a large computer vision Neural-Netwo…
Webinar /Jun 2024
Blog Post /Jun 2025
Blog Post /Jun 2025